Are your DRUG Discovery Teams Playing ‘FAIR’LY
Generating data is resource-intensive, and increasing efforts are made in industry and academia to support analysis of data compendia as well as reuse of existing datasets for purposes different from those for which they were originally generated. These efforts are exemplified by the FAIR principles which provide guidelines for making data “FAIR”: Findable, Accessible, Interoperable, and Reusable. Retrospective data stewardship to FAIRify existing data is extremely time- and cost-consuming, especially for large, long-established pharmaceutical companies that have accumulated considerable amounts of legacy data, often fragmented across the organization. Over time, important information is lost due to organizational changes and employee turnover, either because the data are not well documented or because they are stored in nonstandard or nonmachine-readable formats. Thus, it is crucial to have in place FAIR play processes from the point of data generation, including clear data and metadata management strategies. Figshare can help manage this process.
Organizations must set expectations and provide incentives for scientists generating data to include rich and harmonized metadata, and the process to do so should be simple and straightforward. Tools for metadata capture need to be intuitive, flexible, and configurable enough to handle new data types in real-time, while adhering to established controlled vocabularies and ontologies. Metadata registration and curation tools should capture identifying, descriptive data and relationships in a single source of truth system and seamlessly propagate information to downstream applications and services. User-friendly study design tools should be integrated with both data production and analysis, providing a platform for experimental scientists and data scientists to collaboratively specify, iterate, and agree upon experimental parameters, analysis methods, and statistical power before running studies.
Importantly, clear data access rules need to be established as part of this FAIRification process. The goal of these rules should be to democratize the data, in which they are no longer accessible by a select few but by the whole organization. This requires a cultural shift away from a “my data” and toward an “our data” mindset. These access policies should clearly establish which data can be accessed by whom and when, with a drive towards reducing bureaucracy and speeding up scientific insights. This is especially valuable in drug discovery and development, during which early and broad access to both preclinical and clinical data may enable the generation of new hypotheses or steer existing programs in new directions. Overall, a FAIR data ecosystem is the foundation from which data scientists select and integrate subsets of data necessary to perform meta-analyses across different datasets.
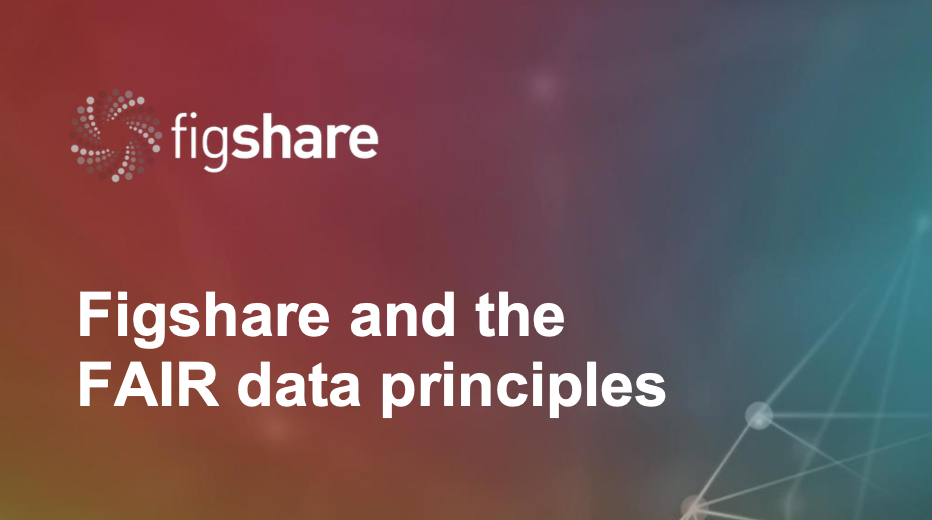
Why FAIR is important to Figshare
One of Figshare’s early goals was to encourage the sharing of negative results and to provide a platform to disseminate those results to reduce duplication of work. Our ethos has not changed and even before the formalisation of the FAIR data principles we believed that data should be findable, accessible, interoperable and reusable. As an extension of those principles we also believe in tracking public research outputs via Altmetrics and Dimensions to further encourage making data openly available. We continue to support academic publishers, preprint platforms, researchers, conferences and labs