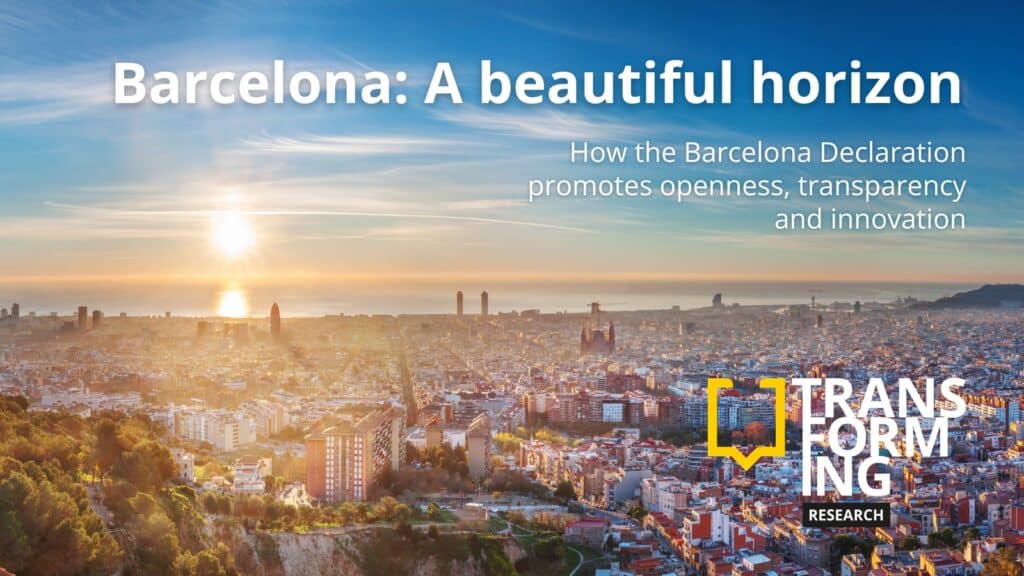
Digital Science welcomes the Barcelona Declaration as a force to continue pushing forward not only openness and transparency but also innovation in and around the scholarly record. Following the launch of this important initiative, we reflect on Digital Science’s path and historical contributions, the economics of maintaining the scholarly record, and its future.
Dimensions is built around open data
In many senses, Dimensions is a demonstration of what can be done when data are made freely and openly available. It would not have been possible to build and maintain Dimensions without the work of initiatives such as I4OC, and the data made available by CrossRef, DataCite, PubMed, ORCID, arXiv and many others. Many pieces of the Dimensions data system leverage use of public sources, and we believe that it is only right and proper to have a version of our product that is available to the community for research purposes at no cost – hence, the free version of Dimensions that we have maintained since 2018, and which we will continue to maintain into the future.
However, service to the community was not the only reason to create a free version of Dimensions back in 2018; it was also about ensuring that researchers had access to search the scholarly record for free and about ensuring that, in an era of increasing research evaluation and increasing research on research, there would be a platform where anyone could go to check results from an analysis or evaluation exercise. At that time, we wrote a paper stating our rationale and principles behind the development of Dimensions and wrote a follow-up piece announcing and committing to continued access for academic research.
In summary, and relevant to current developments, we believe that:
- Researchers have a fundamental right to access research metadata to further their research;
- Research into bibliometrics and scientometrics, and related fields, needs to have a basis for reproducibility and we seek to participate in that ecosystem to ensure that any analysis carried out using Dimensions data is reproducible;
- Data that are used to evaluate academics or institutions should be made available in a way that allows those being evaluated to have an insight into the data on which they are being evaluated.
There is, however, an important additional component that goes beyond these principles – innovation.
A more complex picture
Before we talk about innovation, it is important to acknowledge that Dimensions is not solely built on open data. Indeed, it is a mixed environment with data of different types describing different research objects using different sources. This leads to significant complexity in the data pipeline and in the work that needs to be done to provide “analytics-ready” data. However, for the purposes of the current discussion, it is helpful to understand a bit about the different nature of the sources of data used in our data products. These include open data from open sources. When data are published under a CC0 licence (as Digital Science did with its GRID dataset in 2017) then it is unambiguous that these data may be used in any context, commercial or noncommercial, and that they may be merged with other datasets for the purposes of creating new and better things. It is an interesting question as to whether a Digital Science “mirror” of these helps to make the research infrastructure more robust and easier to access.
Our products also make use of licensed data. These are data for which we have an agreement that restricts its use. Examples can range from research articles; grant data from funders; and, patent documents. They can also include data licensed into products such as Altmetric, which includes data from news providers and social media platforms such as Twitter (X). These data can be expensive to acquire and can only be used and made available in our products within certain limits, even where they are already in the public domain.
All these data and data that are derived from them, even if already freely and openly available, can require substantial resources to compile and process. Examples of such derived data could include funder details, details of ethics statements, conflict of interests, data availability statements, and so on, that Digital Science has transformed, enriched and contextualised. All are activities that take significant investment and add significant value to those who use it. We expect that these types of data will increasingly become part of the Open dataset as the research ecosystem matures. Yet, as we innovate, these are also the data that cost Digital Science the largest investment to produce and maintain, including where this may be done in an automated manner. The infrastructure behind Dimensions is not simply a platform that takes data from open sources and then reserves it for users to consume; rather, it is a complex and expensive mechanism for compiling, refining and improving data so that it can be discoverable, useful and analytics ready.
Taking author contribution statements as an example, the Dimensions team has invested in the creation and curation of AIs that identify author contribution statements across the research literature. These AIs operate at a level of accuracy that still needs improvement, and hence further investment. Neither the scholarly community, nor publishers, nor standards organisations have defined or accepted a standardised data format that makes author contribution statements widely available. As such there is a significant cost to data processing. On top of this, innovations such as the CReDIT taxonomy are neither universally or evenly applied. The use of CReDIT would be of significant value to sociologists who study the research community, as well as to the evaluation community and anyone involved in tenure and promotion processes. And yet, there is no accepted structured data format that makes these data easily available. As such, the Dimensions team is working on the development of a CReDIT data structure and the creation of these data at a level of quality where they can be trusted and used in these important use cases.
As the research ecosystem matures, what should the path from algorithmically generated information back to openly available data with a defined provenance be? One option is to provide enhanced metadata back to publishers to enhance the scholarly record where gaps exist. Arguably, it is not enough for data only to be open – it should be owned by the community that created it, which includes ensuring the context and provenance of the data are maintained. This process has happened many times before, most notably during the application of DOIs to the historical scholarly record.
A model for thinking about innovation
To make sense of this complex landscape we have a mental model that we use to think about the developing world of open research metadata.
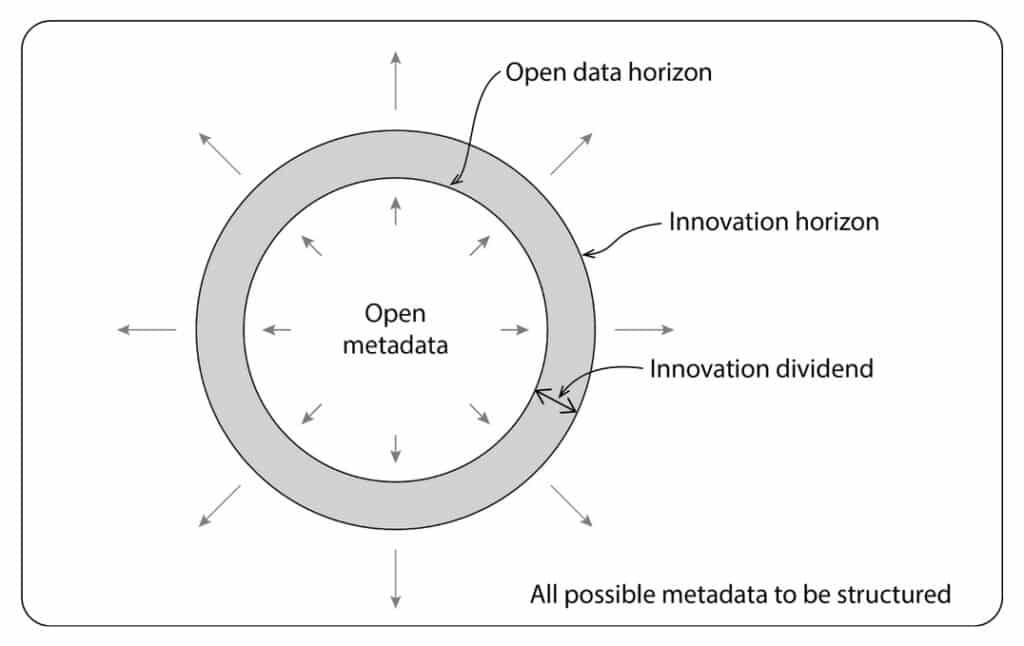
The area outside the outer circle (or horizon) can be thought of as all unpublished articles and all articles as yet unprocessed. With time the outer circle expands encompassing both more detail about the existing published literature (new fields, greater accuracy) and the detail about newly published work. At the horizon of the circle the data are mined and fall inside the circle. The fact that the circle expands is important in this model as the effort to derive the data does not expand proportionally to the volume of data refined, but it does increase. The horizon is representative of the ongoing investment in innovation that is required to derive and improve data from raw, unstructured formats. In practical terms, some cases require humans to identify data from texts; in other cases humans write and train AIs to create annotations and make them available.
The inner circle (or the “beautiful” horizon) can be thought of as open data or data that has become so inexpensive to make available as part of increases in efficiency of the production process that it is completely commoditised. These are data that either cost little to provide or are already refined to the point where little or no innovation is required to make them available. Examples include article title, journal name, page number, DOI and, most recently as a result of I4OC and I4OA, citations and abstracts.
The area between these two circles is where the friction at the heart of the Barcelona Declaration exists. A few years ago, it might be argued that there was no inner circle and yet, over the last 20 years, projects including PubMed, Crossref, I4OC, I4OA and pre-print servers such as RePEc and arXiv have slowly created a space for open data, either through community action or technological progress. Among the contributors to this effort there are some notable players including the Microsoft Academic Search project, 1science from the team at ScienceMetrix, and others.
Such a model is not unusual in other contexts, nor is it surprising that it is the natural point of friction. Determining the time for which an innovation should be profitable and the level of profit is not a trivial problem – it is sometimes left to market forces or sometimes is the result of legislation. In the context of copyright law, which was originally developed to protect creativity, the distance between the circles is determined by law to be 70 years after the death of the author in many geographies, although there are variances. Perhaps closer to home, and less legal (but nonetheless social-contract-style) agreements include humanities PhD theses, which often have an agreed two-year embargo period during which the student has the opportunity to develop and publish a book or otherwise build on top of their work.
There are other non-legislative mechanisms that also determine the distance between analogous horizons in other contexts. One might argue that the creation of a new patented invention is like the innovation horizon of the outer circle, whereas the beautiful horizon of the inner circle is the creation of parallel developments that seek to achieve the same ends as the original invention via different mechanisms. Typically, the time taken for competitors to duplicate an approach, might take several years. At some point the patent will expire, but it may already be rendered useless by the innovations of others.
Perhaps uniquely in the research information sector, Digital Science has pushed both horizons – pushing the innovation horizon:
- Connecting patent data, grant data, clinical trials and datasets in an analytics-ready dataset;
- Pioneering the creation of article level metrics with Altmetric;
- Pioneering the open sharing of research data at scale with Figshare;
- Improving the timeliness of data;
- Innovating to democratise the application of computational power to bibliographic data;
- Showing how to connect bibliographic data to wider contexts to leverage investments in open identifiers;
- Developing the first SDG categorisation;
- Developing transparent categorisation approaches using AI;
- Measuring the progress toward the adoption of OCRID against algorithmically identified research identifiers;
- Making a free ChatGPT experience available that leverages the content in Dimensions;
- Contributing to the ongoing discussions to protect the scholarly record by the open documentation of approaches to identify paper-mill activity;
as well as pushing the open data horizon:
- Making grant data freely available to funders who award less than $1m in funding per year;
- Making Dimensions available for free to Research4Life;
- Making GRID institution data available CC0;
- Making dataset searching and citations freely available in Dimensions and Figshare;
- Making ANZSRC and SDG classification freely available in Dimensions;
- Making grants data available to ORCID profiles through the UberWizard.
Taking a pragmatic position suggests that the annulus needs to be determined dynamically rather than systematically. If an individual or a company invests in pushing the innovation horizon then they are taking a chance on improving the data that researchers and other stakeholders have to make better decisions, gain deeper insights or be more efficient, and there should be an incentive to continue to invest in innovation. If the innovation is incremental or easy to replicate then the returns will be small as others should easily duplicate it. If the innovation is significant then it will be harder for others to reproduce and hence it will take a longer period before competitive forces come to bear.
A step change in technology can upset the equilibrium and change both the current competitive dynamics as well as the future focus of innovation. Machine learning was one of the key technologies that has allowed the Dimensions team to push resources into innovation over the last few years, and enhancements in the AI landscape with large language models (LLMs) will continue to fuel these developments.
At Digital Science, our belief is that by taking risks, being innovative and pushing boundaries, so that clients gain real value and significant benefit from our offerings, there should be an opportunity for an appropriate return on investment. We believe that the chance to profit is naturally kept in check by competition, which typically pushes the outer circle, by initiatives such as the Barcelona Declaration, which often advance the inner circle, and by our own mission as Digital Science to support and serve research and the community around it, where we have clearly demonstrated the ability and the will to move both circles.
The Future
Using the model above, it made sense in the past that scholarly information would be closed. In the 1950s, when Eugene Garfield started the Institute for Scientific Information, the investment required to construct the science citation index was significant. Indeed, it was Garfield’s realisation that 80% of the citations related to 20% of the literature which turned the problem of citation tracking into one that was tractable with technology contemporary to the era.
The investment that needed to be made to “mine” the publication and citation information, given the level and nature of scholarly information infrastructure at this time, was vast. Hence, it is unsurprising that the Science Citation Index was, in essence, the only such index for almost 50 years. With the digitisation of the scholarly record towards the end of the 20th Century, the bar to entry was lowered and PubMed, Crossref, Google Scholar and Scopus were all innovators, introducing competition and, ultimately, creating the Open Data Horizon.
In 2018, Dimensions made use of successive innovations from the community, such as I4OC, together with machine learning to lessen the distance between the two circles.
In the next 10 years, with technological advances in how we write and publish scholarly output, we see a world in which much of the metadata is simply available at the point of production as open data – a true realisation of the Barcelona Declaration. At this point, the distance between the two circles will be zero, with the innovation horizon and the open data horizon coinciding. The effective cost of production of the data will be zero.
So, what will be beyond Barcelona? There are still many challenges regarding research information – there will probably be a further period beyond the Barcelona Declaration’s aims in which, as we already are, we start to invest more heavily in information provenance, the integrity of research information, and in understanding sentiment and bias in the research literature. Our field of focus will shift to ensuring that we can trust the information that will be increasingly important not only in decision making but in forming the basis of AI curricula in the future.
I have confidence that in an innovative field such as research, innovation will continue to be expected of those who seek to serve the space. While Barcelona defines a beautiful horizon, that is still compatible with an endless frontier.