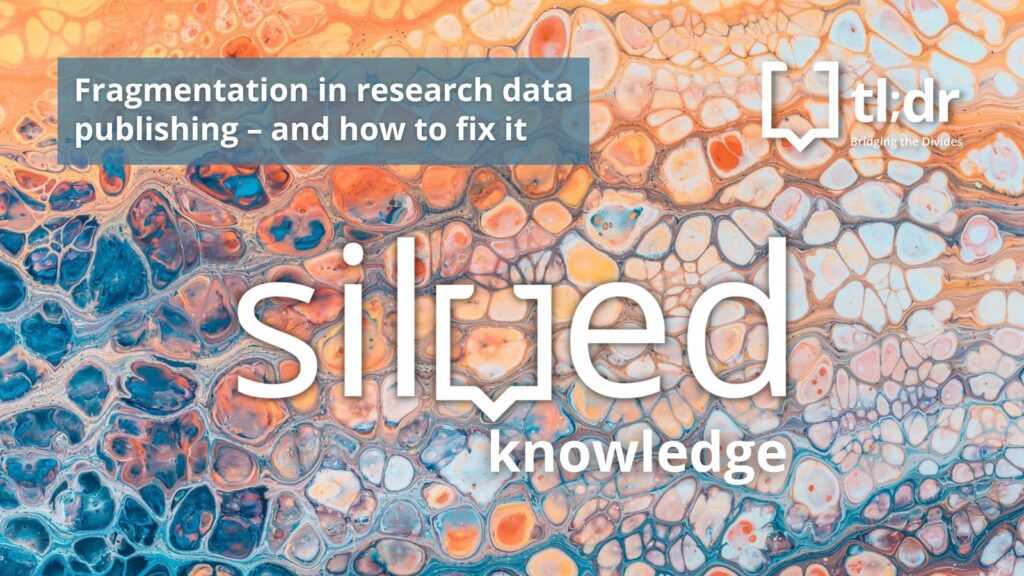
The landscape of open research data is ever-evolving, driven by the relentless advancement of technology and the burgeoning expectations of a data-driven society. This piece navigates research data publication fragmentation, elucidating how the principles of Findable, Accessible, Interoperable, and Re-usable (FAIR) data – are pivotal in this context. FAIR data was a term coined in 2016 that has gained global traction. The 15 points highlighted in Box 2 (below), taken from the original paper show how easily the principles could be differently interpreted by different groups. But surely small differences in interpretation won’t have drastic outcomes, right?
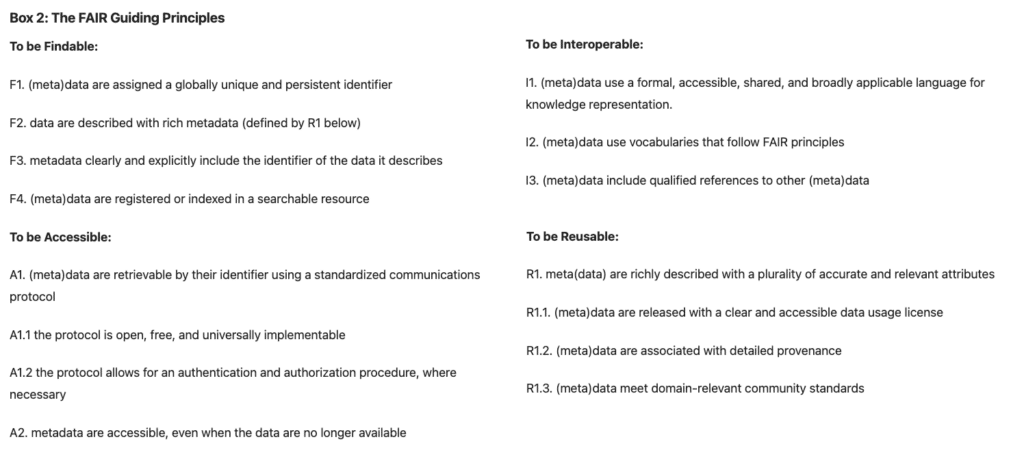
The next paradigm of academic research is AI powered generation of new knowledge. We need to ensure that the algorithms driving the new knowledge are working on normalised and homogenous data and metadata. At Figshare, we produce the State of Open Data each year, in partnership with Springer Nature. The State of Open Data (SOOD) is the longest-running longitudinal study into researchers’ attitudes towards open data. Now in its eighth year, the 2023 survey saw over 6,000 respondents. The report gives us clues as to how consistent approaches to ‘open data’, ‘metadata’ and ‘FAIR data’ are globally. This year’s report highlights that although global trends are useful, now is the time to investigate the nuances at the country and research-area level.
Country and Category
When examining the ten countries with the most SOOD survey respondents, Ethiopia tops the chart in terms of the highest percentage of respondents who ‘strongly support’ a national data mandate, followed by India, Germany, and the United Kingdom. Japan has the lowest percentage of respondents who ‘strongly support’ open data mandates. The idea of mandating more processes for yourself as a researcher, may seem at odds with busy researchers workloads. However, as funder policies become more prevalent in a country, anecdotal evidence suggest that researchers want their peers to be treated equally; “If I have to make my data openly available, everyone should”.
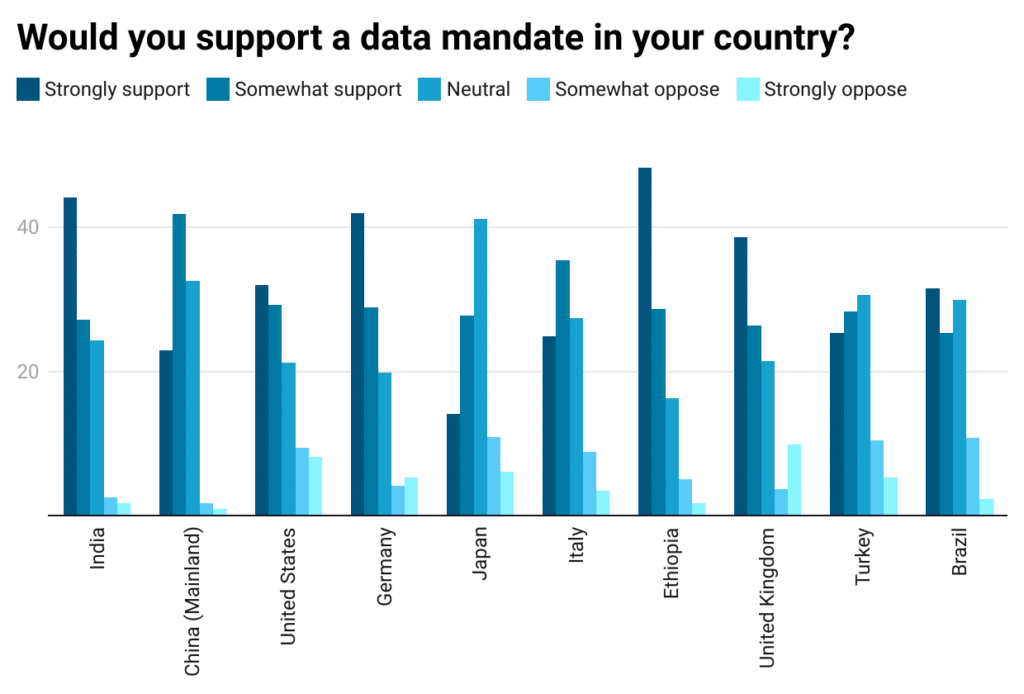
Interestingly, the biggest funder of Ethiopian based publications is the Bill and Melinda Gates Foundation, which has a strong Open Data publishing policy: “Each accepted article must be accompanied by a Data Availability Statement that describes where any primary data, associated metadata, original software, and any additional relevant materials can be found.”
The biggest funder of Japanese based publications is Japan Society for the Promotion of Science (JSPS). The second biggest Funder is Japan Science and Technology Agency, which does ‘require Open Data archiving’ and since 2017 has required funded researchers to develop a data management plan (DMP) defining how to manage research data, and to manage data accordingly. However the graph below shows Japanese researchers have the lowest awareness when it comes to DMPs. This demonstrates the many points at which a country specific, or funder specific open data plan, may struggle to have an impact. In order to start rectifying these discrepancies, we recommend that funders;
- Engage with researchers and institutions to understand specific needs and challenges within their context and tailor open data policies accordingly. Establish advisory committees, consult with experts and collaborate with scientific organisations.
- Establish clear policies that recognize and reward researchers for sharing data openly and integrate these contributions into career progression evaluations
- Ensure that funded projects allocate resources and time for data management, and provide clearer guidelines on data sharing requirements.
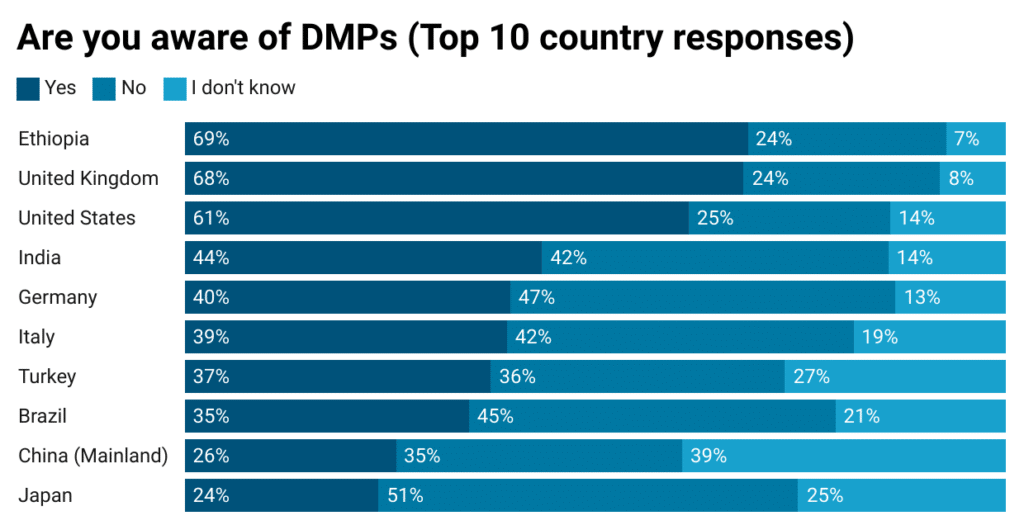
Similarly, different research areas are being onboarded to open practices at different rates. Some research areas are more computational and some research areas have come about in a digital-native setting. A great example of one such case is the academic and financial success of the human genome project. There are also some fields that are the natural leaders when it comes to immediate potential for AI. One such example is Materials Science, driven by The Materials Project – an initiative that harnesses the power of AI and machine learning to accelerate materials discovery and design. So the fact that our survey had the vast majority of materials science researchers unaware of DMPs again paints a worrying picture about mismatches and silo’d opinions in research.
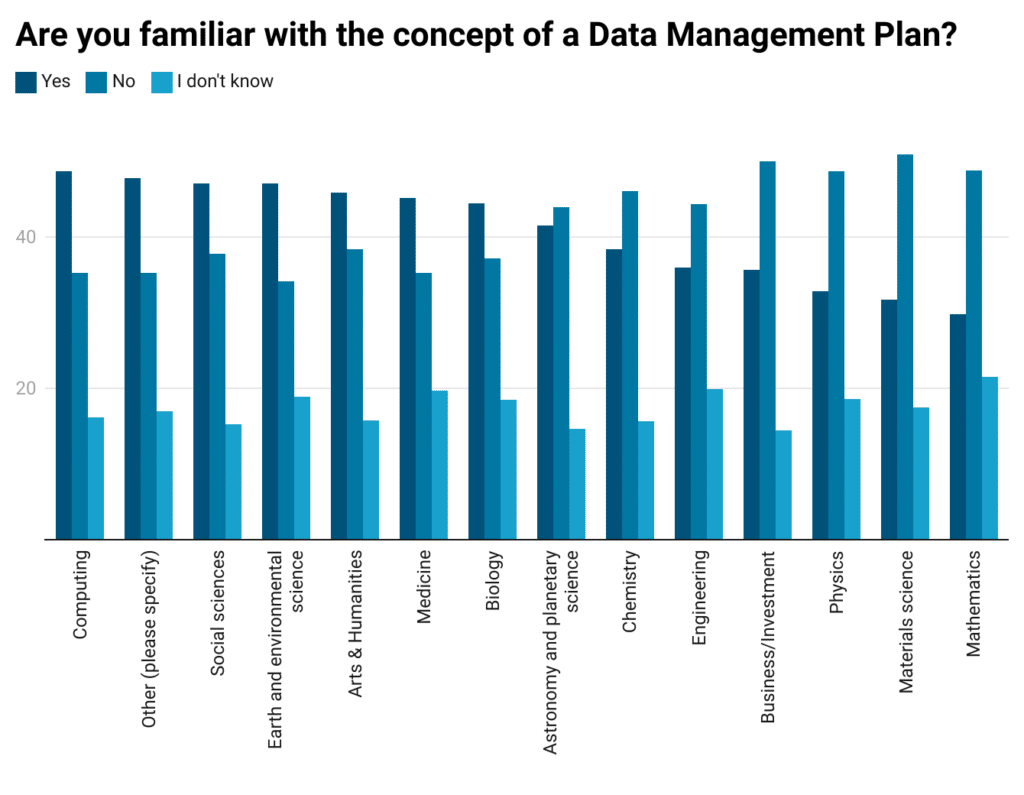
The Chinese Academy of Sciences Computer Network Information Center has launched a partner State of Open Data report that looks at the challenges and opportunities in Chinese academic data publishing. Interestingly, one area they highlight as a concern is the IP of said academic data. In some countries, there is a strong focus on the commercial potential of research. This can lead to reluctance to share data that might be commercially valuable. In contrast, other countries might prioritise open science and public access to research. Some governments might encourage open access and data sharing through mandates and funding, while others might impose restrictions due to national security concerns or to control the flow of information.
Metadata and Openness
Research data is rapidly becoming the energy that will drive big leaps forward in the research. In a time when all of the low hanging fruit in research has been picked. Larger volumes of FAIR data can be processed by machines much more efficiently than humans. Processing this information to infer trends and predicted models allows human expertise to leverage information at a much faster rate, leading to new knowledge. We are in the ‘low hanging fruit’ phase of research powered by algorithms, compute and FAIR data. This author is optimistic that transparency in the code that powers AI will be a requirement of the academic community, particularly when generating new knowledge in healthcare, or fields that impact humanity directly.
There are 2 further, major blockers to get past the low hanging fruit phase. These are the levels of openness of research data and the metadata quality. This is a multi-dimensional problem that draws upon regional differences, subject differences and institutional differences. Pharmaceutical companies will over time benefit from open research data generated by public funding, without the need to contribute in return. Thus, commercial entities may end up with richer data sources, from which to build upon. Likewise, the gulf between subject specific repositories like genbank (single file formats with very descriptive subject specific curated metadata) and generalist repositories (uncurated generic metadata schemas) means that we may have metadata quality-base tiers of academic data. We may also have silos of knowledge which favour those who top up their models with in-house, closed data. Significantly, the private sector, including pharmaceutical companies, have a much more consistent approach when it comes to metadata requirements. One of the benefits of the top-down managed commercial sector over the bottom up, individualistic approach of academia.
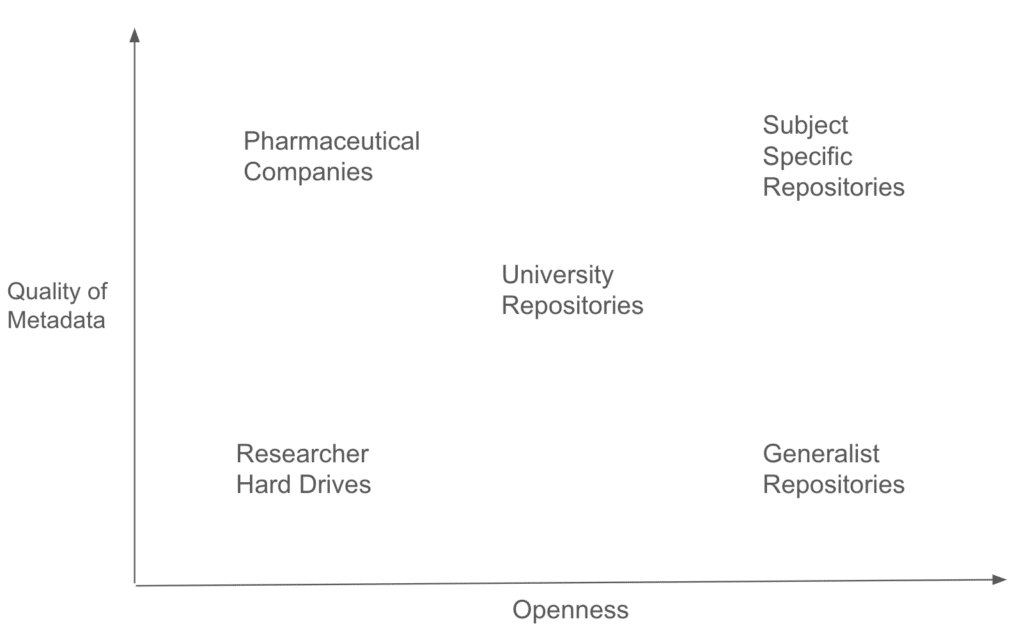
There are strong motivators for pharmaceutical companies to share data transparently. Doing so can improve public perception and trust. This is particularly important in sensitive areas like vaccine development or treatments for major diseases. However, organisations like OpenTrials have demonstrated some of the reluctance to give up any competitor advantages. We also see in the chart above that the destination for research data can produce consistency problems. Some research areas, or even filetypes, are lucky to be supported by subject specific repositories. At Figshare, in line with community best practices, we advise researchers to publish in subject specific repositories where possible. If there are none available, then your University data repository (if they have one), is the next best bet – and finally a generalist repository. The reason for this recommendation is that the support for researchers, and subsequent metadata quality is highest in subject-specific repositories. As it is unreasonable to expect funders to provide a subject-specific repository for every research type – in order to move more research data to the upper right quadrant of the above chart, we should encourage more resources for generalist repositories, or provide thematic repositories. That is, data repositories based around a specific subject, with subject specific metadata. This is a step up from generalist repository use.
As the research data opportunity for low hanging research advances, progress may be delayed whilst we try to normalise the approach at the subject, country and organisational-type level. We should however celebrate the progress that has been made in the last decade. There is a huge amount to be gained by addressing the fragmented set up we currently have. Fortunately, we have a solid framework with global buy-in in FAIR. We are moving forward in good open data publishing from all angles, from funding to training. As such, research data has a monumental opportunity to become un-fragmented in a way that the traditional publication may never get to!